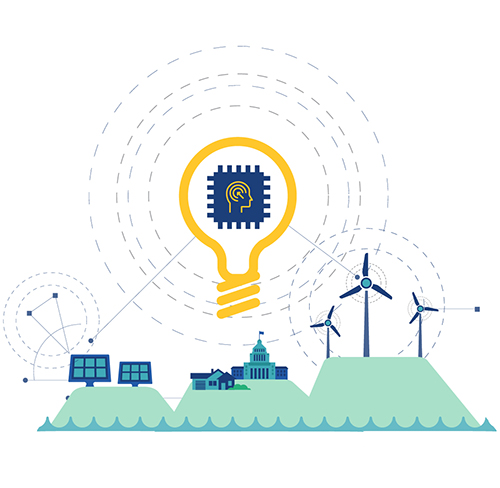
22 7月 2022
Insight
Australia
What role will machine learning, a subset of AI, play in Australia's transition to a zero carbon energy future, and what are some of the implications and trade-offs that come with its increased use in our energy infrastructure?
The rise of renewables
Over the past decade, renewable energy consumption has grown globally at an average annual rate of 13.7%. In Australia, 2020 saw more than a quarter of the country’s total electricity generation coming from renewable sources for the first time. Tasmania, the Australian island state, currently runs on 100% renewable energy. This is good news for climate change. However, integrating these renewable energy sources into existing electricity networks poses challenges. A possible solution are artificial intelligence (AI) technologies, which are becoming increasingly sophisticated at the same time as the growth in renewable energy. |
![]() |
Applying AI to renewable energy technologies
![]()
|
Over the next 10-15 years, more intermittent distributed energy sources (such as wind and solar) will come online as dispatchable centralised generators such as coal are retired. This will require a delicate balancing act to match supply with demand without collapsing the grid. Machine learning technologies can help tackle this challenge.
Machine learning uses algorithms to analyse huge data sets to identify trends and patterns. The algorithm is tasked with making predictions about a target variable, based on the ‘rules’ programmed into the algorithm and the data it receives. The algorithm will seek to identify patterns in the data, use those findings to modify its ‘rules’, and optimise to better undertake further analysis, i.e. the algorithm learns as it works.
Machine learning technologies are increasingly being used in climate science to improve climate modelling and track the effects of climate change.
In the electricity sector, machine learning can also be applied to improve the way energy is distributed and used in the grid. With more renewable energy sources coming online all the time, utilities need better ways of predicting how much energy is needed, in real time and over the long term. Algorithms already exist that can forecast energy demand, but they could be improved by taking into account finer local weather and climate patterns or household behaviour.
Resilience and reliability
![]()
|
The electricity grid must be able to meet customer needs, with minimal power interruptions. When disruptions occur, the grid needs to be able to quickly recover.
Instead of waiting for grid assets to break down, AI is being used to predict problems before they occur. Given the interconnected nature of an electric grid such as the National Electricity Market in Australia, preventing just one equipment failure can avoid colossal cascading blackouts, such as the blackouts in South Australia in 2016. Utilities and generators are using algorithms that analyse industry-wide early failure rates for equipment in order to predict the probability of failure.
Decentralised generation
![]()
|
The distributed nature of many renewable energy sources, such as rooftop and solar is also creating challenges for the grid. An increasingly renewable energy grid has many moving parts, requiring coordination, forecasting and optimization to keep it in balance. Utilities are struggling to manage distributed resources, many of which are not owned, managed, or even visible to the grid managers.
AI software can help to enable utilities to direct electricity arriving into the grid to where it is most needed. However, if machine learning is going to play a greater and more integral role in our energy systems, utilities, policy makers and regulatory bodies need to start thinking about what role they want to play in a much more decentralised energy grid.
A new role for utilities?
The patchwork of distributed energy producers will still need coordination and management. These advancements require a shift in thinking from legacy models of capital investment in a few large energy generation assets to demand management of a growing number of privately owned assets. The protection of customer data and privacy and ensuring cybersecurity of grid management will also need to be considered.
This could mean a new role for utilities as they face a shrinking pool of customers purchasing electricity as more homes and businesses become energy producers themselves. Utilities may have to decide whether to work with software companies to implement AI technologies in their business, or whether they become software companies in their own right.
What are the implications and trade-offs?
Some herald AI technologies as a silver bullet solution to all manner of complex and pressing human challenges from tackling food security to climate change.
What are some of the implications and trade-offs that come with the increased use of machine learning models in our energy infrastructure?
Environmental cost
It is somewhat ironic that for all its potential to reduce electricity usage and support the mass take up of renewable energy generation, machine learning models can also be a large consumer of energy.
Training an algorithm can use vast amounts of computing power. For example, natural language processing (a machine learning technique that helps machines interpret and generate text) is especially power-hungry. Training a single large natural language processing model may consume as much energy as a car over its entire lifetime—including the energy needed to build it1.
If artificial intelligence is to be used to support the mass adoption of renewable energy power generation, we need to ensure that the negative environmental impacts of artificial intelligence are outweighed by its positive ones.
![]()
|
To do this, greater transparency and disclosure of the environmental impact of artificial intelligence technologies, particularly machine learning models, is critical2.
Steps are being taken to address this. The Allen Institute of AI has proposed a certification for AI practices, labelling carbon-neutral AI as “green” and non-carbon-neutral AI as “red” in a bid to help companies navigate this complex area. Research is also being carried out to reduce the carbon footprint of machine learning models.
Data stewardship
Another significant issue that arises across all applications of artificial intelligence is the privacy implications of mass data collection and sharing.
With the widespread adoption of smart grid infrastructure, utilities and network operators have unprecedented levels of consumer data. As machine learning processes rely on vast amounts of data to achieve more accurate and reliable results, data sharing will be critical to successfully implementing machine learning driven technologies in the energy sector.
However, this gives rise to privacy concerns about the information that can be gleaned about an individual from that data and the potential for accidental or malicious surveillance, profiling, behaviour tracking, or even identity theft.
This places additional responsibilities primarily on utilities as guardians of this data and raises important legal questions about customer consent, and the storage, use, transfer, ownership and disposal of customer data.
Ethical issues
Algorithmic decision making is not neutral. There is increasing concern from those working in proximity to the technology sector around bias in algorithmic decision-making and the opacity of the decision making process.
![]()
|
While the primary goal of smart grids is to avoid energy scarcity, balancing this goal against competing priorities will become a source of political and societal debate. For example, how should AI prioritise energy distribution between domestic uses, industrial uses and electric car uses, particularly in cases of energy scarcity? These are not the types of questions that can be resolved by AI alone.
If energy companies and governments are to use AI to make important decisions in our critical national infrastructure, it is imperative that there is public trust in the system.
In order to build public trust, those who are using AI systems must be able to understand and explain why the algorithm made a particular decision and be accountable for the consequences of these decisions. This means remaining open to criticism, disclosing unknowns, and allowing for fixing up issues with poor training data.
We wrote an article about the Australian Human Rights Commission’s recent report on Human Rights and Technology. The report looks closely at the implications of the use of decision making artificial intelligence in the public and private sector in Australia and highlights potential areas of law reform to ensure decision making is “lawful, transparent, explainable, used responsibly, and subject to human oversight, review and intervention”3. Although much of the current guidance on the ethical use of artificial intelligence around the world is not legally binding, energy companies adopting machine learning technologies will be well advised to keep abreast of the fast moving regulatory landscape in their jurisdiction and follow best-practice guidance on how v models should be designed and deployed.
Conclusion
The Australian Energy Market Operator (AEMO), has recognised the opportunities for AI and machine learning in this space and the need to keep pace with the private sector, which has being embracing machine learning technology for some time. In its 2021 corporate plan AEMO commits to developing “new capabilities enabled by technology such as artificial intelligence and machine learning in order to develop strategic forecasting capabilities” over the next three years.
This commitment is welcome. We are at a moment of systemic change in the energy industry with the opportunity to build the foundations of a clean energy future. AI technologies have a meaningful role to play as well as the potential to reshape the operation of the energy market more broadly.
We are at a moment of systemic change in the energy industry with the opportunity to build the foundations of a clean energy future. AI technology is undoubtedly a driving force behind this transition. However, careful consideration must be given to how such technology is designed and deployed and its impact on society to ensure that holistically, we are building a better future for generations to come.
- Melanie Ehrenkranz, How to Train Artificial Intelligence That Won’t Destroy the Environment, OUTLINE (Nov. 4, 2019), h
- Australian Human Rights Commission, Human Rights and Technology (Final Report, 2021)
- E.Strubell, A.Ganesh, A.McCallum, “Energy and Policy Considerations for Deep Learning in NLP” College of Information and Computer Sciences University of Massachusetts Amherst, 5 June 2019
Key contacts
Legal Notice
The contents of this publication are for reference purposes only and may not be current as at the date of accessing this publication. They do not constitute legal advice and should not be relied upon as such. Specific legal advice about your specific circumstances should always be sought separately before taking any action based on this publication.
© Herbert Smith Freehills 2024
Stay in the know
We’ll send you the latest insights and briefings tailored to your needs